- Home
- Editorial
- News
- Practice Guidelines
- Anesthesiology Guidelines
- Cancer Guidelines
- Cardiac Sciences Guidelines
- Critical Care Guidelines
- Dentistry Guidelines
- Dermatology Guidelines
- Diabetes and Endo Guidelines
- Diagnostics Guidelines
- ENT Guidelines
- Featured Practice Guidelines
- Gastroenterology Guidelines
- Geriatrics Guidelines
- Medicine Guidelines
- Nephrology Guidelines
- Neurosciences Guidelines
- Obs and Gynae Guidelines
- Ophthalmology Guidelines
- Orthopaedics Guidelines
- Paediatrics Guidelines
- Psychiatry Guidelines
- Pulmonology Guidelines
- Radiology Guidelines
- Surgery Guidelines
- Urology Guidelines
Using Artificial Intelligence to Deliver Personalized Radiation Therapy
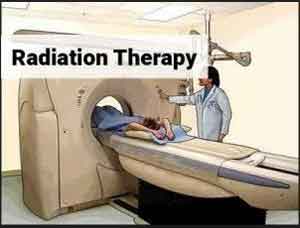
CLEVELAND: New Cleveland Clinic-led research shows that artificial intelligence (AI) can use medical scans and health records to personalize the dose of radiation therapy used to treat cancer patients.
Published today in The Lancet Digital Health, the research team developed an AI framework based on patient computerized tomography (CT) scans and electronic health records. This new AI framework is the first to use medical scans to inform radiation dosage, moving the field forward from using generic dose prescriptions to more individualized treatments.
Currently, radiation therapy is delivered uniformly. The dose delivered does not reflect differences in individual tumor characteristics or patient-specific factors that may affect treatment success. The AI framework begins to account for this variability and provides individualized radiation doses that can reduce the treatment failure probability to less than 5 percent.
“While highly effective in many clinical settings, radiotherapy can greatly benefit from dose optimization capabilities,” says lead author Mohamed Abazeed, M.D., Ph.D., a radiation oncologist at Cleveland Clinic’s Taussig Cancer Institute and a researcher at the Lerner Research Institute. “This framework will help physicians develop data-driven, personalized dosage schedules that can maximize the likelihood of treatment success and mitigate radiation side effects for patients.”
The framework was built using CT scans and the electronic health records of 944 lung cancer patients treated with high-dose radiation. Pre-treatment scans were input into a deep-learning model, which analyzed the scans to create an image signature that predicts treatment outcomes. Using sophisticated mathematical modeling, this image signature was combined with data from patient health records – which describe clinical risk factors – to generate a personalized radiation dose.
“The development and validation of this image-based, deep-learning framework is exciting because not only is it the first to use medical images to inform radiation dose prescriptions, but it also has the potential to directly impact patient care,” said Dr. Abazeed. “The framework can ultimately be used to deliver radiation therapy tailored to individual patients in everyday clinical practices.”
There are several other factors that set this first-of-its-kind framework apart from other similar clinical machine learning algorithms and approaches. The technology developed by the team uses an artificial neural network that merges classical approaches of machine learning with the power of a modern neural network. The network determines how much prior knowledge to use to guide predictions about treatment failure. The extent that prior knowledge informs the model is tunable by the network. This hybrid approach is ideal for clinical applications since most clinical datasets in individual hospitals are more modest in sample size compared to non-clinical datasets used to make other well-known AI predictions (i.e. online shopping or ride-sharing).
Additionally, this framework was built using one of the largest datasets for patients receiving lung radiotherapy, rendering greater accuracy and limiting false findings. Lastly, each clinical center can utilize their own CT datasets to customize the framework and tailor it to their specific patient population.
“Machine learning tools, including deep learning, are poised to play an important role in healthcare,” says Dr. Abazeed. “This image-based information platform can provide the ability to individualize multiple cancer therapies but more immediately is a leap forward in radiation precision medicine.”
This study, which was done in collaboration with Siemens Healthcare, was funded by a National Institutes of Health grant to Dr. Abazeed, the National Cancer Institute, American Lung Association, Siemens Healthcare and VeloSano (Cleveland Clinic’s flagship philanthropic initiative) to advance cancer research.
For more details click on the link: DOI:https://doi.org/10.1016/S2589-7500(19)30058-5
Disclaimer: This site is primarily intended for healthcare professionals. Any content/information on this website does not replace the advice of medical and/or health professionals and should not be construed as medical/diagnostic advice/endorsement or prescription. Use of this site is subject to our terms of use, privacy policy, advertisement policy. © 2020 Minerva Medical Treatment Pvt Ltd