- Home
- Editorial
- News
- Practice Guidelines
- Anesthesiology Guidelines
- Cancer Guidelines
- Cardiac Sciences Guidelines
- Critical Care Guidelines
- Dentistry Guidelines
- Dermatology Guidelines
- Diabetes and Endo Guidelines
- Diagnostics Guidelines
- ENT Guidelines
- Featured Practice Guidelines
- Gastroenterology Guidelines
- Geriatrics Guidelines
- Medicine Guidelines
- Nephrology Guidelines
- Neurosciences Guidelines
- Obs and Gynae Guidelines
- Ophthalmology Guidelines
- Orthopaedics Guidelines
- Paediatrics Guidelines
- Psychiatry Guidelines
- Pulmonology Guidelines
- Radiology Guidelines
- Surgery Guidelines
- Urology Guidelines
New AI system diagnoses neurological diseases on CT in seconds
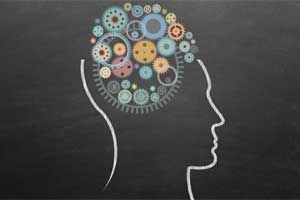
The researchers at the Icahn School of Medicine have developed an artificial intelligence platform that can help in the identification of a range of acute neurological illnesses including hydrocephalus, stroke, and hemorrhage in CT scans in just 1.2 seconds, faster than human diagnosis.
Results of the study, published in the journal Nature Medicine has the potential to triage radiology workflow, thus decreasing time to treatment and improving outcomes.
Eric Oermann, Instructor in the Department of Neurosurgery at the Icahn School of Medicine at Mount Sinai, and colleagues demonstrated a 3D-CNN (convolutional neural networks) architecture that performs weakly supervised classification to screen head CT images for acute neurologic events.
“With a total processing and interpretation time of 1.2 seconds, such a triage system can alert physicians to a critical finding that may otherwise remain in a queue for minutes to hours,” said Oermann. “We’re executing on the vision to develop artificial intelligence in medicine that will solve clinical problems and improve patient care.”
Also Read: Easy bedside test for diagnosing neurological diseases
This is the first study to utilize artificial intelligence for detecting a wide range of acute neurologic events and to demonstrate a direct clinical application.
The researchers used 37,236 head CT scans to train a deep neural network to identify whether an image contained critical or non-critical findings. The platform was then tested in a blinded, randomized controlled trial in a simulated clinical environment where it triaged head CT scans based on severity. The computer software was tested for how quickly it could recognize and provide notification versus the time it took a radiologist to notice a disease. The average time for the computer algorithm to preprocess an image, run its inference method, and, if necessary, raise an alarm was 150 times shorter than for physicians to read the image.
This study used “weakly supervised learning approaches,” which built on the research team’s expertise in natural language processing and the Mount Sinai Health System’s large clinical datasets. Dr. Oermann says the next phase of this research will entail enhanced computer labeling of CT scans and a shift to “strongly supervised learning approaches” and novel techniques for increasing data efficiency. Researchers estimate the goal of re-engineering the system with these changes will be accomplished within the next two years.
“The expression ‘time is brain’ signifies that rapid response is critical in the treatment of acute neurological illnesses, so any tools that decrease time to diagnosis may lead to improved patient outcomes,” says study co-author Joshua Bederson, Professor and System Chair for the Department of Neurosurgery at Mount Sinai Health System.
“The application of deep learning and computer vision techniques to radiological imaging is a clear imperative for 21st-century medical care,” says study author Burton Drayer, the Charles M. and Marilyn Newman Professor and System Chair of the Department of Radiology for the Mount Sinai Health System.
This study was performed by the Mount Sinai AI Consortium, known as “AISINAI”—a group of scientists, physicians, and researchers dedicated to developing artificial intelligence in medicine that will improve patient care and help doctors accurately diagnose disease.
For more information log on to https://doi.org/10.1038/s41591-018-0147-y
Disclaimer: This site is primarily intended for healthcare professionals. Any content/information on this website does not replace the advice of medical and/or health professionals and should not be construed as medical/diagnostic advice/endorsement or prescription. Use of this site is subject to our terms of use, privacy policy, advertisement policy. © 2020 Minerva Medical Treatment Pvt Ltd