- Home
- Editorial
- News
- Practice Guidelines
- Anesthesiology Guidelines
- Cancer Guidelines
- Cardiac Sciences Guidelines
- Critical Care Guidelines
- Dentistry Guidelines
- Dermatology Guidelines
- Diabetes and Endo Guidelines
- Diagnostics Guidelines
- ENT Guidelines
- Featured Practice Guidelines
- Gastroenterology Guidelines
- Geriatrics Guidelines
- Medicine Guidelines
- Nephrology Guidelines
- Neurosciences Guidelines
- Obs and Gynae Guidelines
- Ophthalmology Guidelines
- Orthopaedics Guidelines
- Paediatrics Guidelines
- Psychiatry Guidelines
- Pulmonology Guidelines
- Radiology Guidelines
- Surgery Guidelines
- Urology Guidelines
New algorithm that accurately predicts life expectancy in HF patients
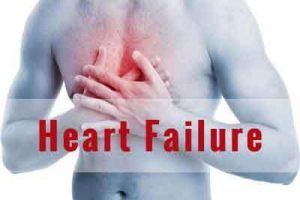
UCLA researchers led by Mihaela van der Schaar, Chancellor’s Professor of Electrical and Computer Engineering at the UCLA Samueli School of Engineering have developed a new algorithm that accurately predicts which people will survive heart failure, and for how long, whether or not they receive a heart transplant. This would enable doctors to make more personalized assessments of people who are awaiting heart transplants, which in turn could enable health care providers to make better use of limited life-saving resources and potentially reduce health care costs. The study has been published in PLOS One.
As precision medicine gains ground in health care, this study could be a key step toward tailoring organ transplant procedures to individual patients.
The algorithm, which the researchers call Trees of Predictors, uses machine learning — meaning that computers effectively “learn” from additional new data over time. It takes into account 53 data points — including age, gender, body mass index, blood type and blood chemistry — to address the complex differences among people waiting for heart transplants and the compatibility between potential heart transplant recipients and donors.
Trees of Predictors uses these 53 data points to predict how long people with heart failure will live, based on whether they receive a transplant or not. (Of those data points, 33 relate to information about the recipients or potential recipients, 14 pertain to the donors and six apply to the compatibility between donor and recipient.)
The researchers tested the Trees of Predictors on 30 years of data on people who were registered with the United Network for Organ Sharing, the nonprofit organization that matches donors and transplant recipients in the U.S. They found that their algorithm provided significantly better predictions for how long someone would live for than the current methods that health care providers use.
It also outperformed predictions from machine learning methods that have been developed by other research groups.
“Our work suggests that more lives could be saved with the application of this new machine-learning–based algorithm,” said van der Schaar, who also is a Turing Fellow at the Alan Turing Institute in London, and the Man Professor at University of Oxford. “It would be especially useful for determining which patients need heart transplants most urgently and which patients are good candidates for bridge therapies such as implanted mechanical-assist devices.”
The algorithm is able to analyze many possible risk scenarios for potential transplant candidates to help doctors more thoroughly assess people who could be candidates for heart transplants, and it is flexible enough to incorporate more data as treatments evolve.
“Following this method, we are able to identify a significant number of patients who are good transplant candidates but were not identified as such by traditional approaches,” said Dr. Martin Cadeiras, a cardiologist at the David Geffen School of Medicine at UCLA. “This methodology better resembles the human thinking process by allowing multiple alternative solutions for the same problem but taking into consideration the variability of each individual.”
For example, when compared against prediction models that most doctors currently use to project which transplant recipients would live for at least three years after a transplant, a commonly used benchmark, the UCLA algorithm outperformed the models by 14 percent — correctly predicting that 2,442 more heart transplant recipients of the 17,441 who received transplants and lived at least that long after the surgery.
In addition to van der Schaar and Cadeiras, the study’s other primary authors are Jinsung Yoon, a UCLA engineering doctoral student, and William Zame, a UCLA distinguished professor of economics and mathematics. Contributing authors are Dr. Amitava Banerjee of the Farr Institute of Health Informatics Research at University College London, and Ahmed Alaa, a UCLA engineering doctoral student.
Van der Schaar said that the Trees of Predictors algorithm could be used for insights from medical databases and many other types of complex databases. Already, Yoon, Zame and van der Schaar have shown it can work to recognize handwriting and to predict credit card fraud and the popularity of specific news items.
The study was supported by the National Science Foundation and the Office of Naval Research.
Disclaimer: This site is primarily intended for healthcare professionals. Any content/information on this website does not replace the advice of medical and/or health professionals and should not be construed as medical/diagnostic advice/endorsement or prescription. Use of this site is subject to our terms of use, privacy policy, advertisement policy. © 2020 Minerva Medical Treatment Pvt Ltd