AI distinguishes false positive from false negative mammograms
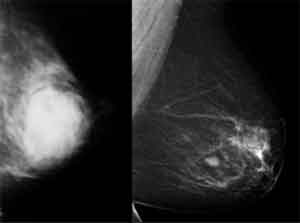
A new study published in the journal Clinical Cancer Research reports that automatic deep learning convolutional neural network (CNN) methods can identify nuanced mammographic imaging features to distinguish recalled-benign images from malignant and negative cases, which may lead to a computerized clinical toolkit to help reduce false recalls.
“In order to catch breast cancer early and help reduce mortality, mammography is an important screening exam; however, it currently suffers from a high false recall rate,” said Shandong Wu, PhD, assistant professor of radiology, biomedical informatics, bioengineering, and clinical and translational science, and director of the Intelligent Computing for Clinical Imaging lab in the Department of Radiology at the University of Pittsburgh, Pennsylvania.
Read Also: 3D mammography more effective for breast cancer screening
“These false recalls resulting in undue psychological stress for patients and a substantial increase in clinical workload and medical costs. Therefore, research on possible means to reduce false recalls in screening mammography is an important topic to investigate,” he added.
Sarah and associates conducted a retrospective study to investigate the revolutionary deep learning methods to distinguish recalled but benign mammography images from negative exams and those with malignancy.
Deep learning convolutional neural network (CNN) models were constructed to classify mammography images into malignant (breast cancer), negative (breast cancer free), and recalled-benign categories. The study included a total of 14,860 images of 3,715 patients from two independent mammography datasets. The ROC curve was generated and the AUC was calculated as a metric of the classification accuracy.
Key study results included are:
- Training and testing using only the FFDM dataset resulted in AUC ranging from 0.70 to 0.81.
- When the DDSM dataset was used, AUC ranged from 0.77 to 0.96.
- When datasets were combined for training and testing, AUC ranged from 0.76 to 0.91.
- When pretrained on a large nonmedical dataset and DDSM, the models showed consistent improvements in AUC ranging from 0.02 to 0.05 compared with pretraining only on the nonmedical dataset.
“We showed that there are imaging features unique to recalled-benign images that deep learning can identify and potentially help radiologists in making better decisions on whether a patient should be recalled or is more likely a false recall. The algorithm’s ability to consistently categorize mammography data shows that AI “can augment radiologists in reading these images and ultimately benefit patients by helping reduce unnecessary recalls,” write the authors.
For reference log on to 10.1158/1078-0432.CCR-18-1115
Disclaimer: This site is primarily intended for healthcare professionals. Any content/information on this website does not replace the advice of medical and/or health professionals and should not be construed as medical/diagnostic advice/endorsement or prescription. Use of this site is subject to our terms of use, privacy policy, advertisement policy. © 2020 Minerva Medical Treatment Pvt Ltd