AI assisted MRI may differentiate between glioblastoma, brain metastasis
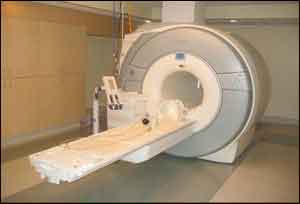
A new study published in the Journal of Magnetic Resonance Imaging reported that differentiation between glioblastoma and brain metastasis showed a high success rate based on postcontrast T1-Weighted MRI.
Differentiation between glioblastoma and brain metastasis is vital due to differing treatment strategies. While MRI is the modality of choice for the assessment of patients with brain tumors, differentiation between glioblastoma and solitary brain metastasis may be challenging due to their similar appearance on MRI.
Read Also: Artificial intelligence may reduce dose of gadolinium in MRI scans
Moran Artzi and associates conducted a retrospective study to differentiate between glioblastoma and brain metastasis subtypes using radiomics analysis based on conventional post‐contrast T1‐weighted (T1W) MRI.
The researchers acquired data from 439 patients: 212 patients with glioblastoma and 227 patients with brain metastasis (breast, lung, and others). The patients had received postcontrast 3D T1W gradient echo images, acquired with 1.5 and 3.0 T MR systems.
Of the patient data, 80% was used for training and 20% was set aside for testing machine-learning algorithms. The analysis included image preprocessing, segmentation of tumor area, and features extraction including the patients' clinical information and tumor location. The researchers calculated the mean sensitivity, specificity, accuracy, and area under the receiver operating characteristic curve (AUC).
Read Also: Artificial intelligence enhances diagnostic accuracy of thyroid cancer: Lancet
The investigators found that the best results for differentiation of glioblastoma from brain metastasis were obtained using the SVM classifier with mean accuracy = 0.85, sensitivity = 0.86, specificity = 0.85, and AUC = 0.96. The best classification results between glioblastoma and brain metastasis subtypes were obtained using SVM classifier with mean accuracy = 0.85, 0.89, 0.75, 0.90; sensitivity = 1.00, 0.60, 0.57, 0.11; specificity = 0.76, 0.92, 0.87, 0.99; and AUC = 0.98, 0.81, 0.83, 0.57 for the glioblastoma, breast, lung, and other brain metastases, respectively.
According to the researchers, their method can be deliberately applicable to classify other brain tumors and evaluate other types of machine-learning classifiers.
"Classification between glioblastoma and brain metastasis subtypes may require additional MR sequences with other tissue contrasts," wrote the authors.
For full information log on to https://doi.org/10.1002/jmri.26643
Disclaimer: This site is primarily intended for healthcare professionals. Any content/information on this website does not replace the advice of medical and/or health professionals and should not be construed as medical/diagnostic advice/endorsement or prescription. Use of this site is subject to our terms of use, privacy policy, advertisement policy. © 2020 Minerva Medical Treatment Pvt Ltd