- Home
- Editorial
- News
- Practice Guidelines
- Anesthesiology Guidelines
- Cancer Guidelines
- Cardiac Sciences Guidelines
- Critical Care Guidelines
- Dentistry Guidelines
- Dermatology Guidelines
- Diabetes and Endo Guidelines
- Diagnostics Guidelines
- ENT Guidelines
- Featured Practice Guidelines
- Gastroenterology Guidelines
- Geriatrics Guidelines
- Medicine Guidelines
- Nephrology Guidelines
- Neurosciences Guidelines
- Obs and Gynae Guidelines
- Ophthalmology Guidelines
- Orthopaedics Guidelines
- Paediatrics Guidelines
- Psychiatry Guidelines
- Pulmonology Guidelines
- Radiology Guidelines
- Surgery Guidelines
- Urology Guidelines
AI may detect clinically meaningful chest X-ray findings at par with radiologists: Radiology study
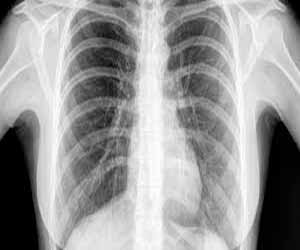
Chest radiography, or X-ray, one of the most common imaging exams worldwide, is performed to help diagnose the source of symptoms like cough, fever and pain. Despite its popularity, the exam has limitations. Deep learning, a sophisticated type of AI in which the computer can be trained to recognize subtle patterns, has the potential to improve chest X-ray interpretation. It has the potential to augment the use of chest radiography in clinical radiology
A sophisticated type of artificial intelligence (AI) can detect clinically meaningful chest X-ray findings as effectively as experienced radiologists, according to a study published in the journal Radiology. Researchers said their findings, based on a type of AI called deep learning, could provide a valuable resource for the future development of AI chest radiography models.
"We've found that there is a lot of subjectivity in chest X-ray interpretation," said study co-author Shravya Shetty, an engineering lead at Google Health in Palo Alto, California. "Significant inter-reader variability and suboptimal sensitivity for the detection of important clinical findings can limit its effectiveness."
Researchers at Google Health developed deep learning models for chest X-ray interpretation that overcome some of these limitations. They used two large datasets to develop, train and test the models. The first dataset consisted of more than 750,000 images from five hospitals in India, while the second set included 112,120 images made publicly available by the National Institutes of Health (NIH).
A panel of radiologists convened to create the reference standards for certain abnormalities visible on chest X-rays used to train the models.
"Chest X-ray interpretation is often a qualitative assessment, which is problematic from a deep learning standpoint," said Daniel Tse, M.D., product manager at Google Health. "By using a large, diverse set of chest X-ray data and panel-based adjudication, we were able to produce more reliable evaluation for the models."
Tests of the deep learning models showed that they performed on par with radiologists in detecting four findings on frontal chest X-rays, including fractures, nodules or masses, opacity (an abnormal appearance on X-rays often indicative of disease) and pneumothorax (the presence of air or gas in the cavity between the lungs and the chest wall).
Radiologist adjudication led to increased expert consensus of the labels used for model tuning and performance evaluation. The overall consensus increased from just over 41 per cent after the initial read to more than almost 97 per cent after adjudication.
The rigorous model evaluation techniques have advantages over existing methods, researchers said. By beginning with a broad, hospital-based clinical image set, and then sampling a diverse set of cases and reporting population-adjusted metrics, the results are more representative and comparable. Additionally, radiologist adjudication provides a reference standard that can be both more sensitive and more consistent than other methods.
"We believe the data sampling used in this work helps to more accurately represent the incidence for these conditions," Dr Tse said. "Moving forward, deep learning can provide a useful resource to facilitate the continued development of clinically useful AI models for chest radiography."
"The NIH database is a very important resource, but the current labels are noisy, and this makes it hard to interpret the results published on this data," Shetty said. "We hope that the release of our labels will help further research in this field."
Journal-Radiology
For further reference log on to :
https://doi.org/10.1148/radiol.2019191293
Disclaimer: This site is primarily intended for healthcare professionals. Any content/information on this website does not replace the advice of medical and/or health professionals and should not be construed as medical/diagnostic advice/endorsement or prescription. Use of this site is subject to our terms of use, privacy policy, advertisement policy. © 2020 Minerva Medical Treatment Pvt Ltd